Note
Go to the end to download the full example code or to run this example in your browser via JupyterLite or Binder
Decision Tree Regression#
A 1D regression with decision tree.
The decision trees is used to fit a sine curve with addition noisy observation. As a result, it learns local linear regressions approximating the sine curve.
We can see that if the maximum depth of the tree (controlled by the
max_depth
parameter) is set too high, the decision trees learn too fine
details of the training data and learn from the noise, i.e. they overfit.
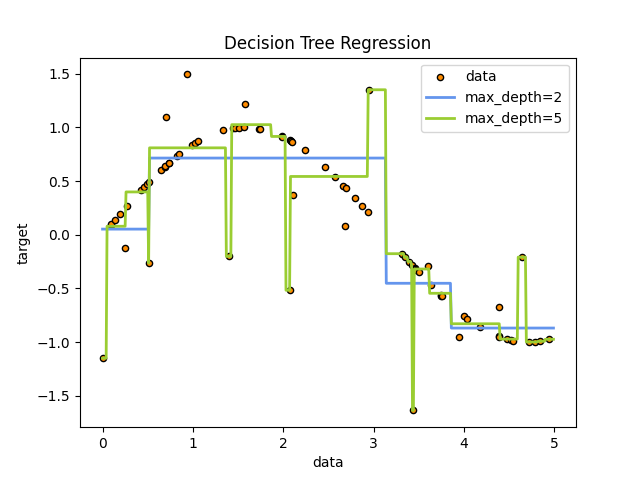
# Import the necessary modules and libraries
import matplotlib.pyplot as plt
import numpy as np
from sklearn.tree import DecisionTreeRegressor
# Create a random dataset
rng = np.random.RandomState(1)
X = np.sort(5 * rng.rand(80, 1), axis=0)
y = np.sin(X).ravel()
y[::5] += 3 * (0.5 - rng.rand(16))
# Fit regression model
regr_1 = DecisionTreeRegressor(max_depth=2)
regr_2 = DecisionTreeRegressor(max_depth=5)
regr_1.fit(X, y)
regr_2.fit(X, y)
# Predict
X_test = np.arange(0.0, 5.0, 0.01)[:, np.newaxis]
y_1 = regr_1.predict(X_test)
y_2 = regr_2.predict(X_test)
# Plot the results
plt.figure()
plt.scatter(X, y, s=20, edgecolor="black", c="darkorange", label="data")
plt.plot(X_test, y_1, color="cornflowerblue", label="max_depth=2", linewidth=2)
plt.plot(X_test, y_2, color="yellowgreen", label="max_depth=5", linewidth=2)
plt.xlabel("data")
plt.ylabel("target")
plt.title("Decision Tree Regression")
plt.legend()
plt.show()
Total running time of the script: (0 minutes 0.099 seconds)
Related examples
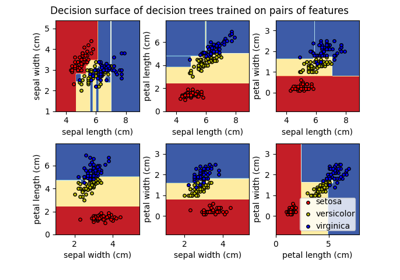
Plot the decision surface of decision trees trained on the iris dataset
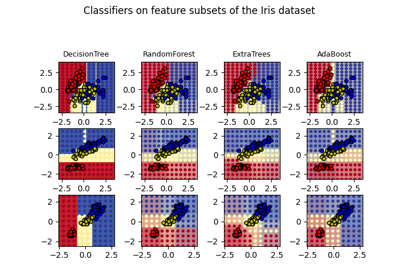
Plot the decision surfaces of ensembles of trees on the iris dataset
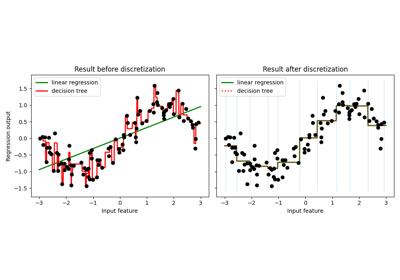
Using KBinsDiscretizer to discretize continuous features